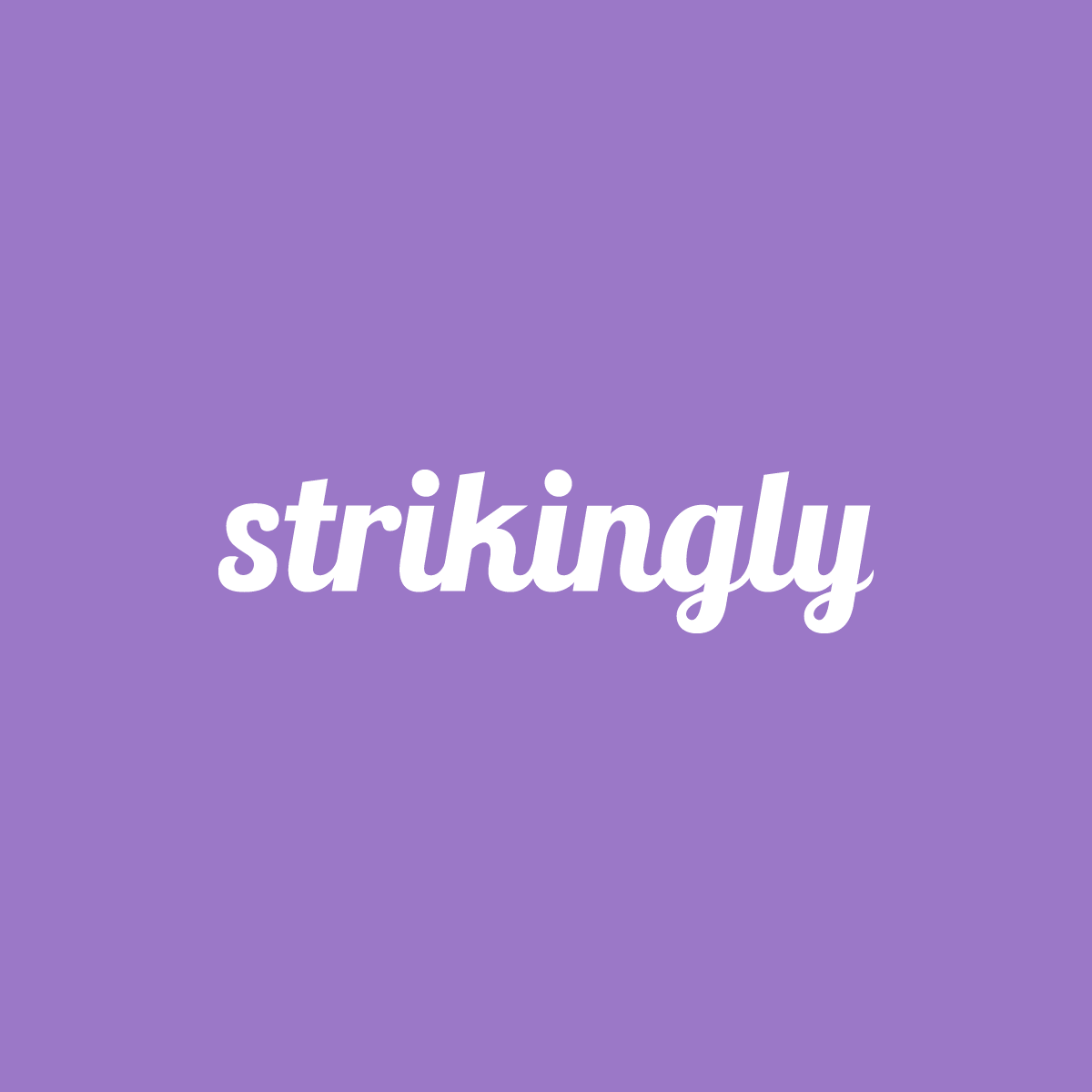
Dr. Pamela Douglas
Dr. Pamela Douglas
Professor Computational Neuroscience
Dr. Pamela Douglas's exceptional research and areas of expertise have garnered significant interest from various sources, leading to numerous grants and funding opportunities that have propelled her work forward. Some of the notable projects she has secured funding for include the Comprehensive Training in Neuroimaging Fundamentals and Applications, supported by the NIH Clinical Center, and the High Throughput Analysis of the ADHD 200 Data Set, funded by the Klingenstein Third Generation Foundation. Additionally, she has received support from organizations such as the W.M. Keck Foundation, the National Science Foundation, and the National Institutes of Health for her research on topics including neuro-engineering, Parkinson's disease modeling, and biomarkers of attention-deficit/hyperactivity disorder. Dr. Douglas's contributions to the field have not gone unnoticed, as her research has been published in esteemed peer-reviewed journals. Her extensive body of work, consisting of 52 publications, provides valuable insights and serves as a guiding light for fellow researchers. Her articles have been featured in renowned publications such as Front Neurosci, Frontiers in Human Neuroscience, Journal of Neuroscience Methods, and Current Opinion in Neurobiology. These publications have garnered thousands of citations, highlighting the impact and significance of her research in the scientific community. As a computational neuroscientist, Dr. Douglas possesses a specific interest in the brain's remarkable ability to organize itself and generate 1/f spectral rhythms that mimic those of the environment. She hypothesizes that this mirroring process serves to reduce the brain's energy requirements for modeling the environment while creating an optimal learning landscape. To explore these concepts, she has utilized various empirical data collection methods, including transcranial ultrasound, fMRI, and EEG. By combining datasets, she develops cognitive computational models and employs pattern classification tools to evaluate representational patterns in fMRI data based on decoding frameworks.
Dr. Pamela Douglas © 2023. All rights reserved.